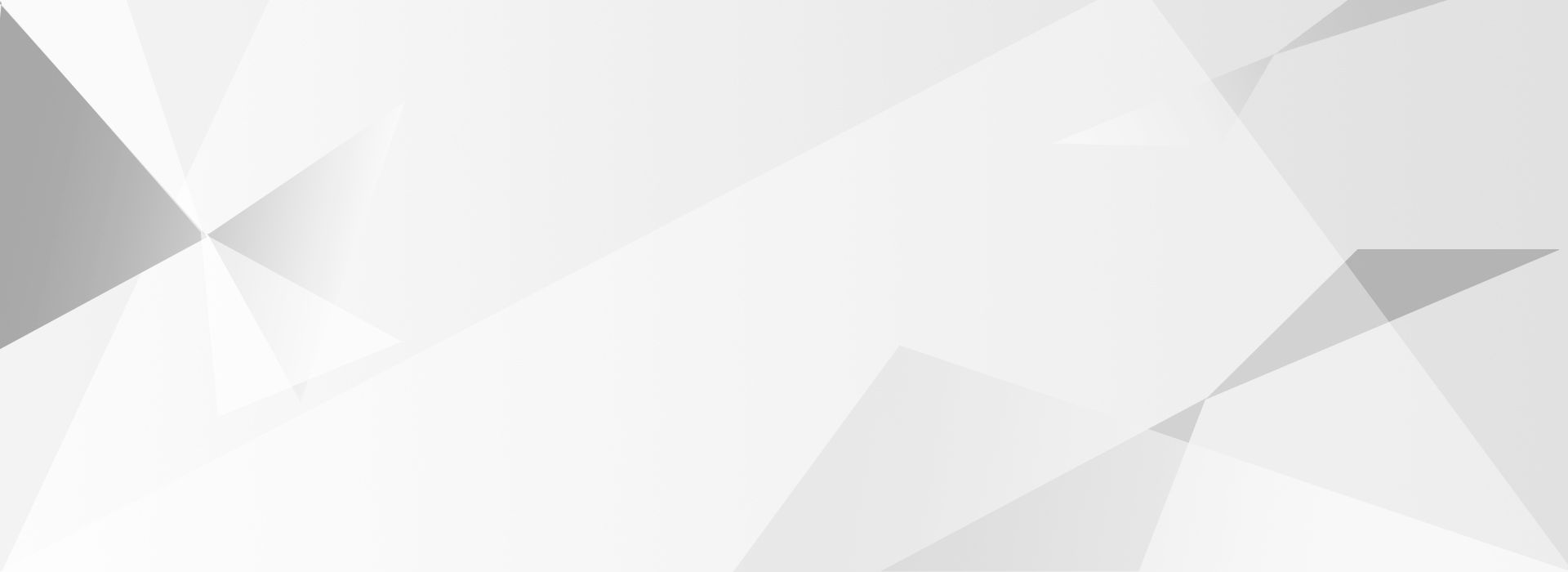
Context-Aware Multi-View Summarization Network for Image-Text Matching
Abstract
​Image-text matching is a vital yet challenging task in the field of multimedia analysis. Over the past decades, great efforts have been made to bridge the semantic gap between the visual and textual modalities. Despite the significance and value, most prior work is still confronted with a multi-view description challenge, i.e., how to align an image to multiple textual descriptions with semantic diversity. Toward this end, we present a novel context-aware multi-view summarization network to summarize context-enhanced visual region information from multiple views. To be more specific, we design an adaptive gating self-attention module to extract representations of visual regions and words. By controlling the internal information flow, we are able to adaptively capture context information. Afterwards, we introduce a summarization module with a diversity regularization to aggregate region-level features into image-level ones from different perspectives. Ultimately, we devise a multi-view matching scheme to match multi-view image features with corresponding text ones. To justify our work, we have conducted extensive experiments on two benchmark datasets, i.e., Flickr30K and MS-COCO, which demonstrates the superiority of our model as compared to several state-of-the-art baselines.
Pipeline
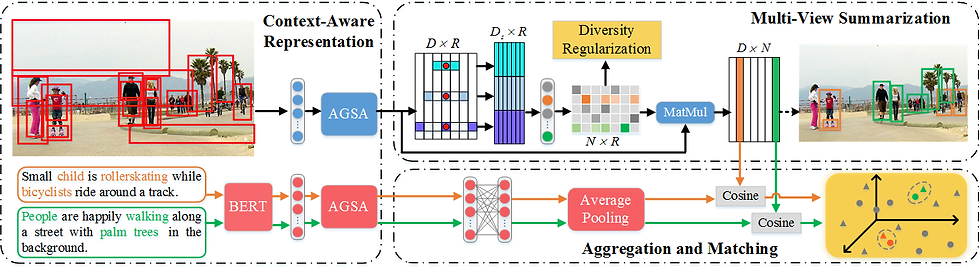
Codes & Data
-
Codes
-
Precomputed Features
-
Pretrained BERT
VSRN
SAEM
CAMERA
Flickr30K & MSCOCO
-
Positions
-
Pretrained CAMERA